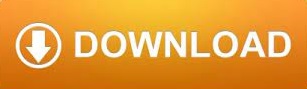

Consequently, the AngelList data supports rejecting the log-normal fit in favor of the power-law fit. The top-performing early-stage investment on AngelList has a return of over 100x a return of this magnitude would be virtually impossible under a log-normal distribution (recall that this is a log-log plot, making the parabolic drop-off in likelihood of the orange line especially severe). The plot shows that the log-normal distribution closely fits the data for smaller multiples before beginning to diverge at around a 10x return multiple. The orange line below shows the log-normal distribution that was fit to the observed spread of our real data: While this distribution has many similar properties to a power law distribution on a log-log plot the log-normal distribution follows a parabolic curve rather than a straight line, with likelihood falling off at right tail of return multipliers.

In a world where startups receive a sequence of well-behaved random percent changes in their valuations (e.g., the company's business improves or falls by 1% each day at random), then it is well-known that company valuations should follow a log-normal distribution. To confirm a power-law fit, we can compare the way it forecasts the distribution of startup valuations against a competing hypothesis. Since both of the axes in the figure are log-scaled, this is colloquially known as a “log-log plot” and is a standard way of showing power-law distributed data. For instance, the red dot at (22, 0.01) indicates that 1% of positive investments have at least a 22x return. The y axis is the log-scaled fraction of positive investments that generate at least that return multiple. The x axis of this plot is the log-scaled return multiplier of the investment (i.e., 1x = money returned without profit, 10x = 900% return). That provides us with a universe of 1,808 investments to examine 1.īelow is a figure of a power-law fit over the subset of those investments that have positive returns (realized or unrealized), before fees, on the platform 2: (The right-hand bound of this figure is truncated at a 100x multiple for obfuscation the fit line is made with untruncated data.)
IKUNA ANGELIST SERIES
To evaluate the distribution of early-stage investment returns, we selected all of the AngelList platform investments prior to Series C that are at least one year old and have a valuation change, or that have already exited. Supporters of the power-law hypothesis point to a handful of anecdotes on portfolio construction, but until now data that could rigorously evaluate the power-law hypothesis in venture capital has been absent. Thiel suggests that, consequently, investors should only invest in “ seven or eight promising companies from which you think you can get a 10x return.” The current venture obsession around counting “unicorns”, investments in companies that have a billion-dollar-plus valuation, probably derives its intellectual justification from this math-driven championing of outliers. In a power law world, the only thing that matters is the presence of a home run investment in the portfolio. Grounded in that experience, Thiel is an enthusiastic proponent of venture capital returns being power-law distributed: where the best investments are worth exponentially more than the great investments, which are themselves worth exponentially more than merely good investments. And the very best early investments offer incredible multiples: Peter Thiel made around $1.1 billion from his half-million dollar early investment in Facebook, a 2,200x return. Analyzing early-stage returnsĮarly-stage investing is an asymmetric bet: you can only lose your investment, but you could gain a multiple of it. The prominence of these huge winners suggests that an indexing strategy of investing in the entire early-stage venture universe will outperform roughly three-quarters of early-stage venture capital funds.
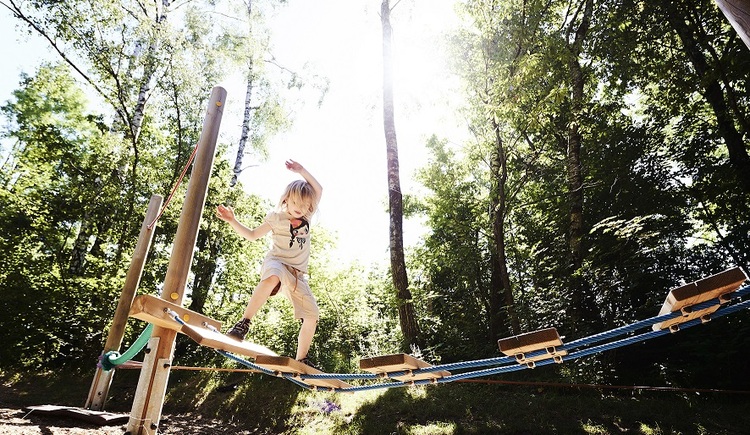
Using AngelList’s curated data we show that the returns of early-stage investments follow a fairly extreme power law distribution, with enormous positive outliers skewing portfolio performance. It takes a top-quartile VC fund to outperform the early-stage venture market.
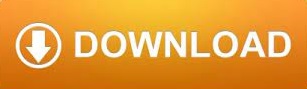